Pilots do something that a lot of non-pilots will find fairly weird if not outright horrifying: We read accident (“crash”) reports. Some of us spend a lot of time reading accident reports, actually. Officially “Accident Reports”, these are put out by the US National Transportation Safety Board (NTSB) after investigation into a crash or an “incident.” In addition to aviation-related reports, there are highway and railroad reports, and even hazardous materials incidents.
Reports come in two flavors, a “preliminary” report, and ultimately, a “final” report after the investigation has completed. The final reports includes such items as conclusions and the probable cause of the accident or incident. To make life easier, they also include a Recommendations section, which, well, includes recommendations for how to keep this type of accident from happening in the future. These tend to be regulatory in nature, as they are geared towards the FAA.
The search form for aviation reports is here–https://www.ntsb.gov/_layouts/ntsb.aviation/index.aspx–if you’re, uh, thinking you want to get into this sort of thing.
Why do pilots do this? The rationale is pretty simple: To learn from the mistakes of others. Or, to learn how a bad day was kept from becoming a worse day after something broke.
What Does This Have to Do With SQL Server?
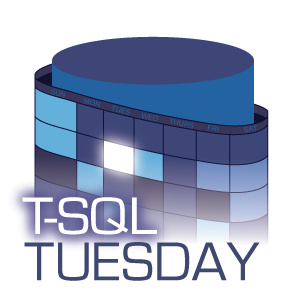
Great question. Besides the fact that I think piloting airplanes and DBA-ing are the same job, just with different scenery, I wish we had this kind of transparency in the IT world when things went wrong. When a corporation has a big security incident, we’re likely not to hear a lot of details publicly about what went wrong and what was done to mitigate similar attacks in the future. This kind of information could help everyone. This is one of the things that cloud providers do quite a bit better: When something breaks, we get good information on what happened, why, and what’s going to be done about it. Of course, this is done because public cloud providers basically have to–if things went down a lot and we never heard why, that provider probably wouldn’t have a lot of customers for very long.
This brings me to T-SQL Tuesday.
Tell me (us all, obviously) about something recently that broke or went wrong, and what it took to fix it. Of course, the intent here would be for this to be SQL Server-related, but it doesn’t have to be. We can all learn from something going wrong in infrastructure-land, or how there was a loophole in some business process that turned around and bit somebody’s arm. It doesn’t even have to be all that recent–maybe you’ve got a really good story about modem banks catching on fire and that’s how you found out the fire suppression system hadn’t been inspected in years. Just spitballin’ here. If you’ve got an incident whose resolution can help someone else avoid the same problem in the future or improve a policy as a preventative measure, let us hear about it.
The Rules
Here are the rules as set out for the T-SQL Tuesday blog party.
- Your post should be published on Tuesday, 14 July, 2020 between midnight and 11:59:59 UTC/GMT/ZULU
- Include the T-SQL Tuesday logo in your post
- Link back to this invitation (usually done through the logo)
(this will get syndicated, so link back to the original on airbornegeek.com, please) - Include a comment on the invitation post or a trackback link
- Enjoy the chance to be creative and share some knowledge.